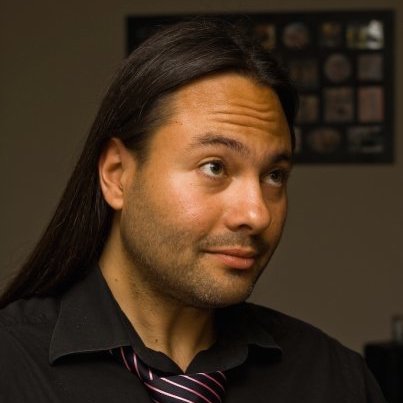 |
Bio: Lorenzo grew up on pizza, spaghetti, and Phrack, first. Underground and academic research interests followed shortly thereafter. He is a Full Professor of Computer Science at UCL Computer Science, where he leads the Systems Security Research Lab in the Information Security Research Group. Lorenzo's research vision focuses on understanding and improving the effectiveness of machine learning methods for systems security in the presence of adversaries. In particular, he investigates the intertwined relationships of program analysis and machine learning and the implications they have towards realizing Trustworthy ML for Systems Security. Lorenzo is Program Co-Chair of the Deep Learning and Security workshop (with IEEE S&P) 2021-22, DIMVA 2021-22, was Program Co-Chair of ACM EuroSec 2019-20 (with ACM EuroSyS), and General Co-Chair of ACM CCS 2019. He holds a PhD in Computer Science from the University of Milan (2008), held Post-Doctoral and Visiting positions at VU Amsterdam (2010-11), UC Santa Barbara (2008-09), and Stony Brook University (2006-08), worked in the Department of Informatics at King's College London (2018-21), where he held the Chair in Cybersecurity (Systems Security), and the Information Security Group at Royal Holloway, University of London (2012-18). He has definitely never stopped wondering and having fun throughout.
|
Transcending Transcend: Revisiting Malware Classification in the Presence of Concept Drift
Thursday, 23 June 2022, Tulla-Hörsaal (CS, 11.40) at 4:00 p.m.
You can also join us via Zoom:
https://kit-lecture.zoom.us/j/62133300729?pwd=cVMvRkNHckxFVHAzOTgvOGdneVZuQT09
Meeting-ID: 621 3330 0729
Kenncode: 693962
Abstract: No day goes by without reading machine learning (ML) success stories across different application domains. Systems security is no exception, where ML's tantalizing results leave one to wonder whether there are any unsolved problems left. However, machine learning has no clairvoyant abilities and once the magic wears off, we're left in uncharted territory. For intsance, machine learning for malware classification shows encouraging results, but real deployments suffer from performance degradation as malware authors adapt their techniques to evade detection. This phenomenon, largely known as concept drift, occurs as new malware examples or, rather, our understanding of their representation, evolve and become less and less like the original training examples. A promising method to cope with this phenomenon is to equip classifiers with a rejection option in which examples that are likely to be misclassified are instead quarantined until they can be expertly analyzed.
In this talk, I will provide a smooth introduction to the field of malware classification leading up to our upcoming IEEE S&P 2022 paper, which proposes TRANSCENDENT. TRANSCENDENT is a rejection framework built around conformal prediction theory that fuels its statistical engine, which equips classifiers with the ability to discern whether examples should be rejected because they will likely be misclassified. Through several case studies, we will see how TRANSCENDENT outperforms state-of-the-art approaches while generalizing across various malware domains and classifiers. These insights support both old and new empirical findings, towards a sound and practical classification with rejection solution. We release TRANSCENDENT as open source, to aid the adoption of rejection strategies by the security community.